Domain-specific AI models are artificial intelligence systems designed to excel in a particular field or industry. Unlike general-purpose AI models that are trained on vast amounts of data across various domains, domain-specific models focus on acquiring deep knowledge and expertise within a specific niche. This specialization enables them to deliver more accurate, efficient, and tailored solutions compared to their general-purpose counterparts.
The importance of tailoring AI to specific domains cannot be overstated. By leveraging domain-specific knowledge, these models can better understand the nuances, complexities, and unique challenges associated with a particular field. This understanding allows them to make more informed decisions, identify patterns and trends that might be missed by general-purpose models, and ultimately provide more valuable insights and outcomes.
However, developing domain-specific AI models also presents unique challenges. Acquiring high-quality, relevant data can be difficult, especially in niche domains with limited public datasets. Additionally, transferring domain expertise into the AI model can be complex, requiring careful consideration of knowledge representation and transfer techniques. Despite these challenges, the potential benefits of domain-specific AI are substantial, and their applications are rapidly expanding across various industries.
Understanding Domain-Specific AI
Domain-specific AI models are characterized by their deep understanding of a particular field or industry. This understanding is achieved through a combination of factors:
- Domain-specific knowledge: These models are trained on datasets that are highly relevant to the target domain. This allows them to acquire a rich understanding of the domain’s concepts, terminology, and underlying principles.
- Specialized algorithms: Domain-specific AI models often employ algorithms and techniques that are specifically designed to address the unique challenges and requirements of their target domain. This can include specialized feature engineering, data preprocessing, and model architectures.
- Knowledge graphs: In many cases, domain-specific AI models incorporate knowledge graphs to represent and reason about domain-specific information. Knowledge graphs provide a structured way to store and connect related concepts, enabling the model to make more informed and accurate inferences.
Building domain-specific AI models is essential for several reasons:
- Improved accuracy and performance: By focusing on a specific domain, these models can achieve higher levels of accuracy and performance compared to general-purpose models. This is because they are better equipped to understand the nuances and complexities of the target domain.
- Enhanced efficiency and cost-effectiveness: Domain-specific AI models can be more efficient and cost-effective to develop and deploy. This is because they can be tailored to specific use cases, reducing the need for unnecessary features or functionality.
- Tailored solutions for specific problems: Domain-specific AI models can provide highly tailored solutions for specific problems within a given domain. This can lead to significant improvements in productivity, efficiency, and decision-making.
- Increased interpretability and explainability: Domain-specific AI models can often be more interpretable and explainable than general-purpose models. This is because they are trained on data that is more closely aligned with human understanding of the domain, making it easier to understand their decision-making processes.
While domain-specific AI offers numerous benefits, its development is not without its challenges:
- Data scarcity and quality: Acquiring high-quality, relevant data for training domain-specific AI models can be a significant hurdle. In many niche domains, data may be limited, biased, or noisy, which can hinder model performance.
- Domain expertise and knowledge transfer: Transferring human domain expertise into an AI model requires careful consideration of knowledge representation and transfer techniques. This can be challenging, especially in complex domains with intricate relationships and dependencies.
- Computational resources and scalability: Developing and deploying domain-specific AI models can be computationally intensive, requiring significant hardware and software resources. This can be particularly challenging for large-scale applications or domains with complex models.
- Ethical considerations and bias mitigation: As with any AI system, domain-specific AI models are susceptible to biases that can lead to unfair or discriminatory outcomes. Addressing these ethical concerns requires careful consideration of data quality, model design, and deployment practices.
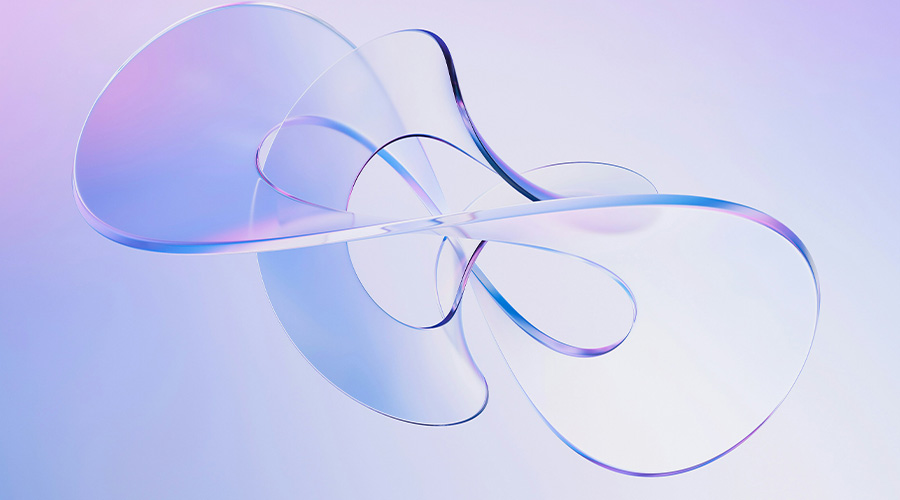
Applications of Domain-Specific AI
Domain-specific AI is finding widespread application across various industries. Here are some notable examples:
Healthcare
- Medical diagnosis: AI models can assist in diagnosing diseases by analyzing medical images, patient data, and genetic information.
- Drug discovery: AI can accelerate drug discovery by identifying potential drug candidates and predicting their efficacy and safety.
- Personalized medicine: Domain-specific AI can help develop personalized treatment plans based on individual patient characteristics and genetic makeup.
Finance
- Fraud detection: AI models can detect fraudulent activities by analyzing patterns in financial data and identifying anomalies.
- Risk assessment: AI can assess financial risk by evaluating factors such as creditworthiness, market trends, and economic indicators.
- Algorithmic trading: AI-powered algorithms can execute trades automatically based on real-time data and market analysis.
Manufacturing
- Predictive maintenance: AI can predict equipment failures before they occur, reducing downtime and maintenance costs.
- Quality control: AI can inspect products for defects and ensure quality standards are met.
- Supply chain optimization: AI can optimize supply chain operations by improving inventory management, transportation planning, and demand forecasting.
Customer Service
- Chatbots: AI-powered chatbots can provide personalized customer support and answer queries efficiently.
- Personalized recommendations: AI can recommend products or services based on customer preferences and behavior.
- Sentiment analysis: AI can analyze customer feedback to understand their satisfaction levels and identify areas for improvement.
Education
- Personalized learning: AI can tailor educational content to individual students’ needs and learning styles.
- Intelligent tutoring systems: AI-powered tutors can provide personalized guidance and support to students.
- Automated grading: AI can automate the grading of assignments and exams, freeing up teachers’ time.
These are just a few examples of the many applications of domain-specific AI. As the technology continues to evolve, we can expect to see even more innovative and impactful uses in the future.
Future Trends and Developments
As domain-specific AI continues to advance, several key trends and developments are expected:
- Hybrid AI models: Combining domain-specific and general-purpose AI models can offer the best of both worlds, leveraging the strengths of each approach. Hybrid models can be particularly effective in complex domains that require a combination of general knowledge and domain-specific expertise.
- Explainable AI: There is a growing emphasis on developing explainable AI models that can provide transparent explanations for their decisions. This is particularly important in domains where trust and accountability are critical, such as healthcare and finance.
- Federated learning: Federated learning enables AI models to be trained on decentralized data, preserving privacy and security. This is particularly relevant for domain-specific AI, where data may be sensitive or distributed across multiple organizations.
- Continuous learning and adaptation: Domain-specific AI models will need to be capable of continuous learning and adaptation to stay relevant in dynamic environments. This will require techniques for handling concept drift, dealing with evolving data distributions, and incorporating new knowledge.
- Ethical considerations and bias mitigation: Addressing ethical concerns and mitigating biases will remain a critical challenge in the development and deployment of domain-specific AI. This will require ongoing research and development, as well as the establishment of ethical guidelines and regulations.
These trends and developments will shape the future of domain-specific AI, leading to even more powerful and impactful applications across various industries. As AI technology continues to evolve, it is essential to address the challenges and opportunities associated with domain-specific AI to ensure its responsible and beneficial use.